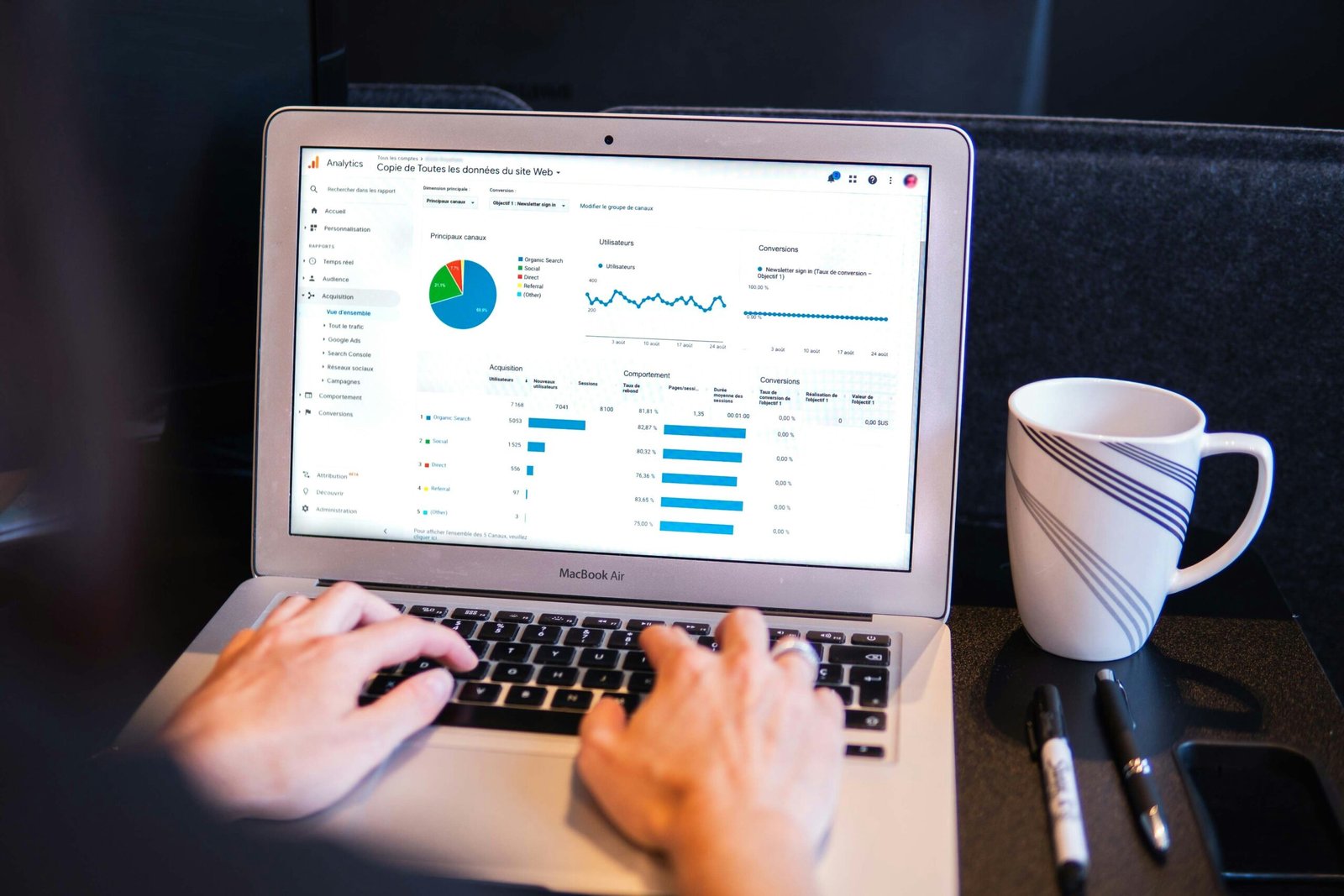
Understanding Data Analysis and Data Science
Data analysis and data science are two interrelated fields that play a crucial role in modern business decision-making. Data analysis refers to the systematic application of statistical and logical techniques to describe, summarize, and compare data. It aims to extract meaningful insights that can inform strategic planning and operational improvements. Businesses utilize data analysis to interpret historical data, identify trends, and support forecasts, thereby enabling more informed choices.
On the other hand, data science encompasses a broader spectrum of activities, integrating domain expertise, programming skills, and knowledge of mathematics and statistics. Data science not only includes data analysis techniques but also focuses on creating algorithms and predictive models to gain actionable insights from complex data sets. It involves the use of advanced analytics and machine learning methodologies, enabling organizations to uncover patterns and make predictions about future trends.
Both data analysis and data science rely heavily on various tools and methodologies. Common tools employed in data analysis include Excel, SQL databases, and business intelligence platforms such as Tableau and Power BI. These tools enable analysts to create visual representations of data and generate reports that facilitate informed decisions. In contrast, data scientists often use programming languages like Python or R, along with libraries and frameworks that support machine learning and statistical analysis, such as TensorFlow and Scikit-learn, to build sophisticated models.
Organizations leverage these practices to enhance performance and maintain a competitive edge. By harnessing data analysis, businesses can gain insights that drive operational efficiency, while data science empowers them to innovate and pursue new opportunities. Ultimately, understanding these concepts will help businesses appreciate their significance and identify ways to implement them effectively for success.
The Role of Data in Business Decision Making
In today’s fast-paced business environment, the importance of data analysis and data science in decision-making processes has become increasingly apparent. Companies are leveraging data to streamline operations, enhance customer experiences, and gain competitive advantages. A data-driven approach to decision-making allows organizations to make informed choices based on empirical evidence rather than gut feelings or outdated practices.
Evidence suggests that businesses that embrace data-driven decision-making witness notable improvements in performance. For instance, the global retail giant Walmart utilizes data analytics for inventory management and forecasting demand. By analyzing sales data, customer preferences, and external factors such as weather patterns, Walmart can optimize its stock levels, reducing waste and enhancing customer satisfaction. This strategic use of data not only drives efficiency but fosters a culture of continual improvement.
Another exemplary case involves Netflix, which heavily relies on data science to inform its content creation and recommendation algorithms. By analyzing viewer preferences and behavior, Netflix can tailor its offerings, leading to unprecedented viewer engagement and retention rates. This reliance on data underscores the necessity for organizations to utilize data analytics effectively, ensuring that their decisions align with consumer interests and market dynamics.
However, the risks of ignoring data insights in business are substantial. Organizations that fail to base their strategies on reliable data may face significant challenges, such as misallocation of resources and missed opportunities. Moreover, cultivating a data-informed culture encourages collaboration across various departments, empowering employees to make decisions backed by data rather than intuition alone.
Implementing a framework for data analysis and science is not merely beneficial; it is essential for businesses seeking to thrive in an increasingly competitive landscape. By embracing data in the decision-making process, organizations can harness its full potential, driving sustained success and innovation.
Implementing Data Analysis and Data Science Strategies
The integration of data analysis and data science into business operations is a multifaceted process that requires careful planning and execution. To begin with, organizations should set clear objectives that define what they aim to achieve through data initiatives. These objectives may involve enhancing operational efficiency, improving customer experiences, or creating predictive models for better decision-making. Establishing measurable goals ensures that the data strategies align with the overall business vision and yield tangible benefits.
After determining objectives, selecting the appropriate tools and technologies becomes crucial. Businesses must conduct thorough research to identify software and platforms that best meet their data analysis requirements. Popular tools such as Tableau for data visualization or Python and R for statistical analysis can be instrumental in carrying out effective data science strategies. Moreover, leveraging cloud-based solutions can provide scalability and flexibility, accommodating varying data sizes and analysis needs.
Equally important is assembling a skilled team that can proficiently handle data initiatives. This may involve hiring data scientists, analysts, and IT professionals who collaborate to collect, clean, analyze, and interpret data. Furthermore, investing in training for existing employees can be beneficial, as it promotes a data-driven culture within the organization. It is essential to create an environment that encourages continuous learning to stay updated with the latest trends and technologies in data science.
However, businesses may encounter challenges in the implementation phase, including resistance to change, data privacy concerns, and insufficient infrastructure. To address these issues, it is vital to foster open communication regarding the benefits of data analysis and data science. Additionally, establishing a robust data governance framework can mitigate privacy risks, while ongoing evaluation of tools and processes can ensure that the infrastructure remains capable of supporting data strategies effectively.
Measuring Success and Future Trends
In the realm of data analysis and data science, measuring success is essential for businesses striving to enhance their performance. One of the primary methods for gauging the impact of data strategies is through the establishment and monitoring of key performance indicators (KPIs). These KPIs serve as quantifiable metrics that allow companies to assess the effectiveness of their data initiatives. Common KPIs include customer acquisition costs, customer retention rates, and return on investment (ROI) from data-driven projects. By analyzing these indicators, organizations can determine whether they are harnessing data effectively to drive growth and profitability.
Moreover, it is crucial for businesses to continuously evaluate their data strategies in relation to these KPIs to ensure alignment with their overall objectives. Regular assessments can lead to informed adjustments, optimizing strategies to better meet customer needs and market demands. The adoption of advanced analytics tools further enhances this capacity, enabling organizations to derive insights from vast data sets and pinpoint areas for improvement in real-time.
Looking ahead, several emerging trends in data analysis and data science are set to revolutionize the business landscape. The integration of artificial intelligence (AI) and machine learning (ML) into data processes is rapidly gaining traction. These technologies facilitate more sophisticated analyses, enabling businesses to uncover intricate patterns and trends that were previously undetectable. As companies increasingly leverage predictive analytics, the ability to anticipate future customer behavior and preferences will enhance competitiveness.
Additionally, the rise of big data and automation will continue to redefine how organizations operate. By embracing these changes, businesses can streamline their processes, improve decision-making, and enhance overall agility. It is imperative that companies stay ahead of these trends to effectively harness the power of data, ensuring sustained success in an ever-evolving market landscape.